AI for autonomous driving
With AI in autonomous driving (AD) at the helm, autonomous vehicles are turning science fiction into reality. At Vention, we make this industry U-turn by building AI-powered automotive solutions that deliver cutting-edge innovation, enhance fuel efficiency, and provide seamless integration.
AI for autonomous vehicles: stats and trends
The automotive software market is accelerating with no signs of slowing down in the next decade. Valued at $19 billion in 2023, it’s expected to reach $32.2 billion by 2030 at a CAGR of 7.8 percent.
This growth is sparked by rising interest in advanced driver assistance systems (ADAS) and autonomous vehicles (AV), driven by breakthrough AI technologies.
Revenue
Revenue
Expected revenue
Expected revenue
Statista projects the automotive intelligence market to surge from $38.5 billion in 2024 to $74.5 billion by 2030.
Revenue
Revenue
Projected market size
Projected market size
By 2035, the autonomous driving sector could generate $300 to $400 billion in revenue, offering lucrative opportunities for original equipment manufacturers (OEMs), suppliers, and tech providers.
Leading industry players are responding to these trends with new initiatives and investments.
Volkswagen, for example, launched its first autonomous vehicle testing program in Austin in 2023, aiming for a commercial launch by 2026. In March 2023, Ford Motor Company established Latitude AI to develop hands-free, eyes-off-the-road automated driving systems.
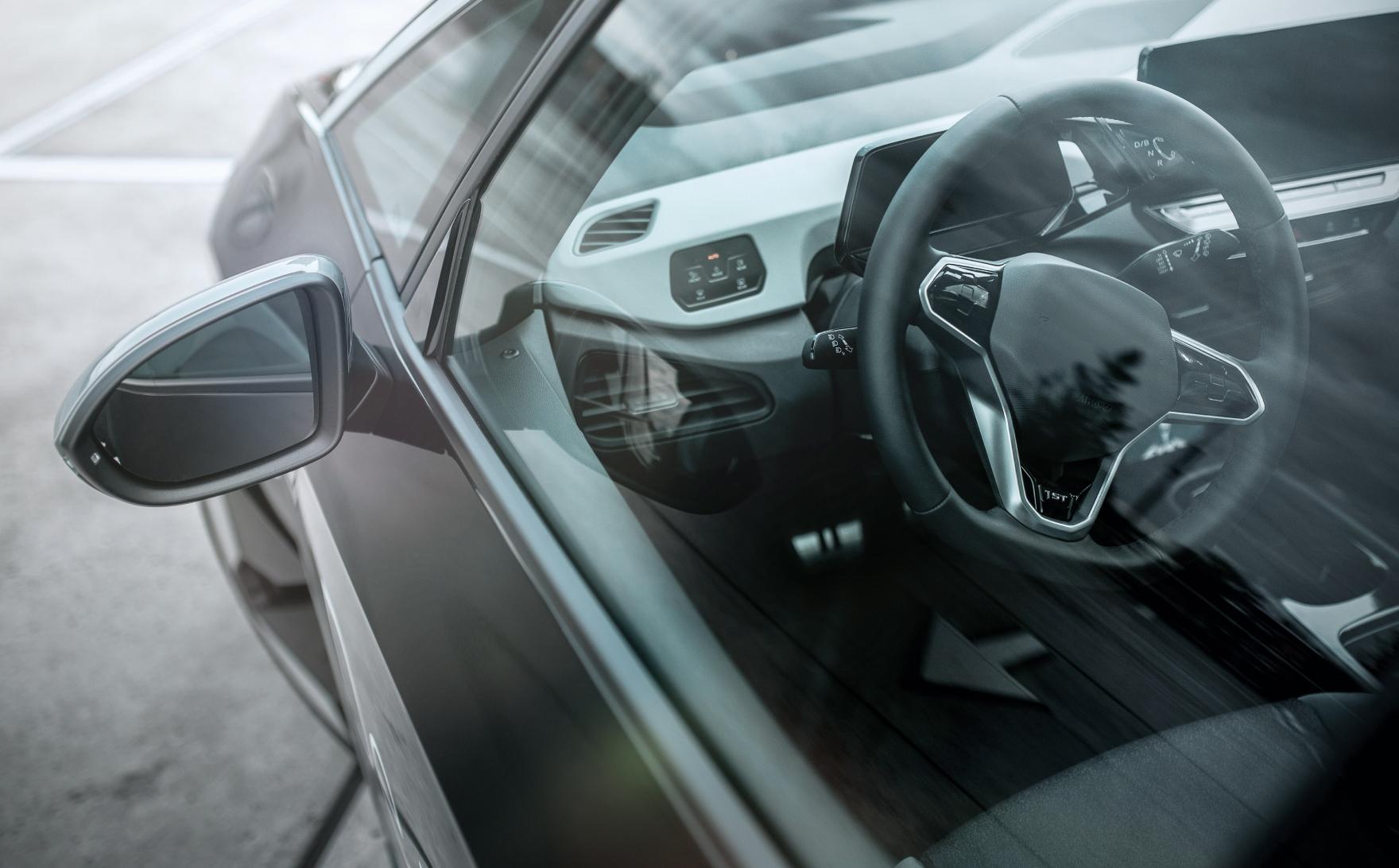
Autonomous driving: What's under the hood
Self-driving cars incorporate a medley of technologies to create an interconnected system capable of navigating roads with minimal human intervention.
The thinker: artificial intelligence and machine learning
As autonomous driving relies entirely on AI/ML technology that mirrors human decision-making, it couldn't exist without AI. AVs use ML algorithms to process inputs from diverse onboard sensors and cameras, allowing the vehicle to understand its surroundings. This process not only recognizes moving objects, but also forecasts potential environmental changes.
The ability to anticipate and respond to situations safely sets AI in self-driving cars apart from more basic forms of automation.
The watcher: sensors and cameras
Autonomous vehicles come equipped with various sensors that provide comprehensive feedback on their surroundings.
-
Light detection and ranging (LiDAR) sensors excel at creating 3D maps by emitting light pulses and measuring their return time after hitting nearby objects.
-
Radar uses radio waves to detect the distance and velocity of objects around the vehicle in different weather conditions.
-
Cameras offer the visual acuity needed to capture detailed information, such as road signs, traffic lights, road markings, and other drivers' behavior.
Together, these sensors do more than see — they perceive. Providing overlapping and complementary data ensures autonomous vehicle systems have redundant information to make split-second driving decisions.
The interpreter: computer vision
Computer vision (CV) technology integrated into automotive software allows vehicles to interpret cues similarly to human drivers. Using advanced image processing algorithms, AVs can recognize traffic signs, signals, lane markings, and gestures from other drivers or pedestrians. This capability is crucial for navigating complex traffic situations and complying with local driving rules.
The navigator: GPS
Armed with detailed mapping data, GPS technology plots routes for autonomous vehicles with remarkable precision. The navigation system ensures the chosen path is always the most efficient by considering variables like real-time traffic flow, road conditions, and construction updates.
The synthesizer: sensor fusion
In the high-stakes environment of road travel, having reliable data is key. Sensor fusion combines information from various sensors to create a cohesive, unified, and more dependable perception of the environment.
This process enhances sensory accuracy and minimizes information gaps, ensuring that if one sensor fails, others can compensate, maintaining full coverage of the area around the vehicle.
The communicator: connectivity
Leveraging vehicle-to-everything (V2X) communication, autonomous vehicles' understanding of the environment extends beyond the immediate sensors embedded in the cars. This technology allows vehicles to exchange data with —essentially, 'talk' to— each other, road infrastructure, and even pedestrians’ smart devices.
Each vehicle becomes part of a larger network, boosting awareness and enhancing safety through collective intelligence.
The executor: control systems
Sophisticated sensors and AI analytics are ineffective without the ability to act. This is where control systems translate complex data into precise steering, braking, and accelerating movements.
These systems are the final link in the chain, engineered to carry out decisions with utmost precision and ensure that the vehicle reacts appropriately to real-time traffic conditions.
The validator: simulation and testing platforms
Before real-world deployment, autonomous vehicles undergo extensive trials within virtual environments. By replicating diverse driving scenarios, including potential hazards, these fail-safe testing platforms guarantee that each system performs optimally and reacts appropriately under extreme conditions.
The role of artificial intelligence in autonomous vehicles
Core components of AI in autonomous vehicles
Machine learning and deep learning
Machine learning and deep learning (DL) form the bedrock of autonomous driving intelligence. These technologies enable automotive software to process colossal volumes of information from sensors and cameras for object detection, behavior prediction, and appropriate responses. ML algorithms are trained on vast datasets to identify patterns and predict outcomes.
Deep learning (DL) in autonomous driving — a more advanced form of ML — excels at handling a broader array of data sources, including unstructured data, requiring minimal human intervention and delivering more accurate outcomes. By utilizing neural networks, DL processes data through multiple layers of neurons that progressively identify intricate data characteristics.
Neural networks
If ML/DL is the brain of autonomous driving, neural networks are its neurons. Modeled after the human brain's structure, these networks are adept at handling massive amounts of sensory data and discovering intricate relationships within that data.
The layered architecture of neural networks enables autonomous vehicles to precisely interpret their environment, facilitating essential functions like object detection, lane recognition, and pedestrian identification. For example, an initial neuron layer might detect an object with a specific shape, and based on that information, a subsequent layer could recognize the object as a stop sign.
Computer vision
Another key AI technology at the core of autonomous driving is computer vision. Like human eyes, CV enables cars to "see" the environment and make sense of the visual world.
By leveraging computer vision, self-driving vehicles can interpret traffic signals, read road markings, and monitor the movement of surrounding objects. This comprehension is crucial for safe road trips, enabling vehicles to respond to changing traffic conditions, detect hazards, and make calibrated driving decisions in real time.
Data analysis and decision-making
Driving requires continuous focus, rapid reactions, and making immediate choices. The technology to replace human drivers must excel in these areas, and AI is up to the task. AI systems make thousands of crucial decisions per mile, such as stopping for pedestrians or adjusting speed for changing road conditions.
By using a combination of neural network-based systems to recognize complex driving scenarios and rule-based logic for specific responses through if-then rules, vehicles can safely navigate intersections, dodge obstacles, and adapt to unexpected situations efficiently and consistently.
Learning and adaptation
The ability of ML algorithms to continuously learn and adapt in response to new data and experiences is foundational to intelligent automotive software and a primary rationale for autonomous driving. Unlike most humans, who may be reluctant to learn from past mistakes, AI thrives on learning and refining, becoming more efficient and safer with continued use.
Continuous updates and retraining of ML models keep autonomous vehicle software sharp. This helps it adapt to changing road conditions, emerging technologies, and unforeseen challenges, delivering unmatched reliability.
Safety and reliability
Safety and reliability are table stakes in driving, and AI for autonomous vehicles plays a crucial role in ensuring both. AI automotive software is designed to handle various scenarios using redundant systems and fail-safes to prevent accidents. By constantly monitoring the environment and adapting to changing conditions, whether sudden obstacles or adverse weather, AI ensures that autonomous vehicles are a viable and secure mode of transportation.
However, critics argue that AI systems are still vulnerable, advocating for AI to face licensing requirements like vision and performance tests for pilots and drivers to ensure robust and reliable autonomous driving systems.
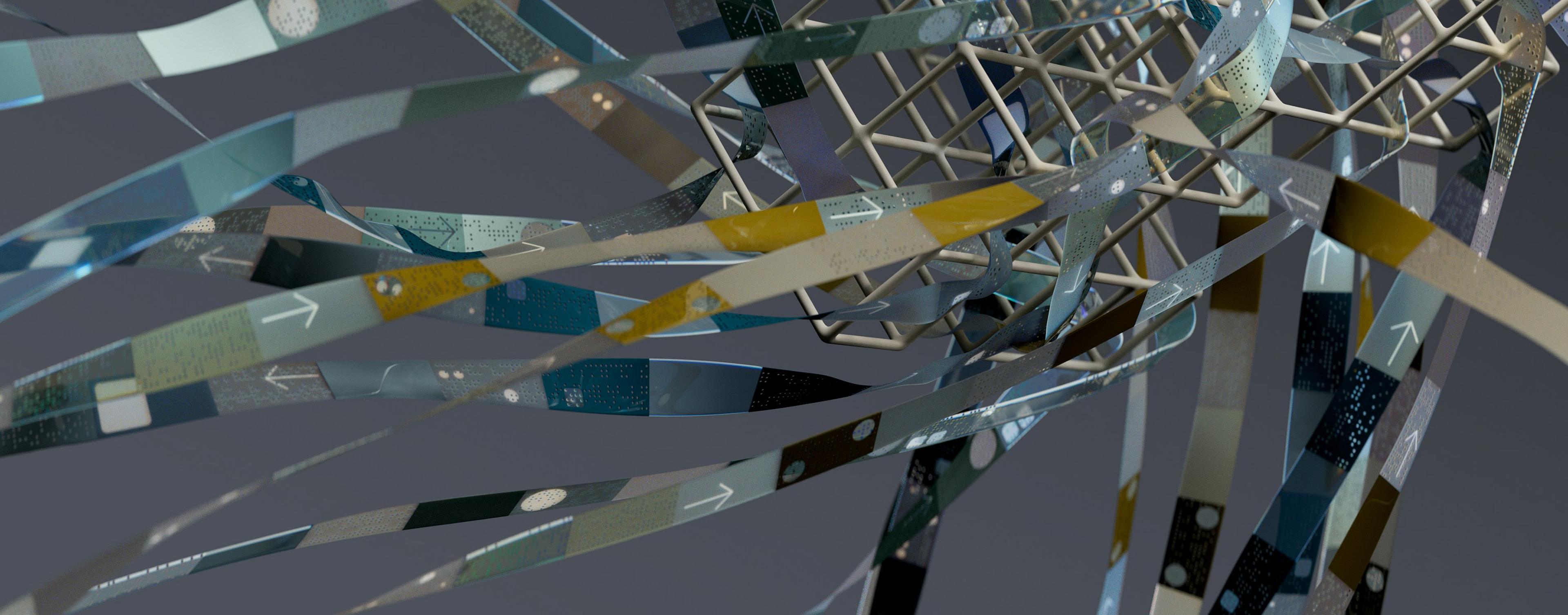
Ready to hit the road to a driverless future?
Speak with our experts today to learn how our AI solutions can navigate your autonomous journey.
Six real-life examples of AI in self-driving cars
Although fleets of driverless cars may not fill the roads soon, real-world applications of artificial intelligence in self-driving cars are bringing the driverless future closer. AI addresses long-standing challenges related to autonomous vehicles' engineering, procurement, manufacturing, and lifecycle services.
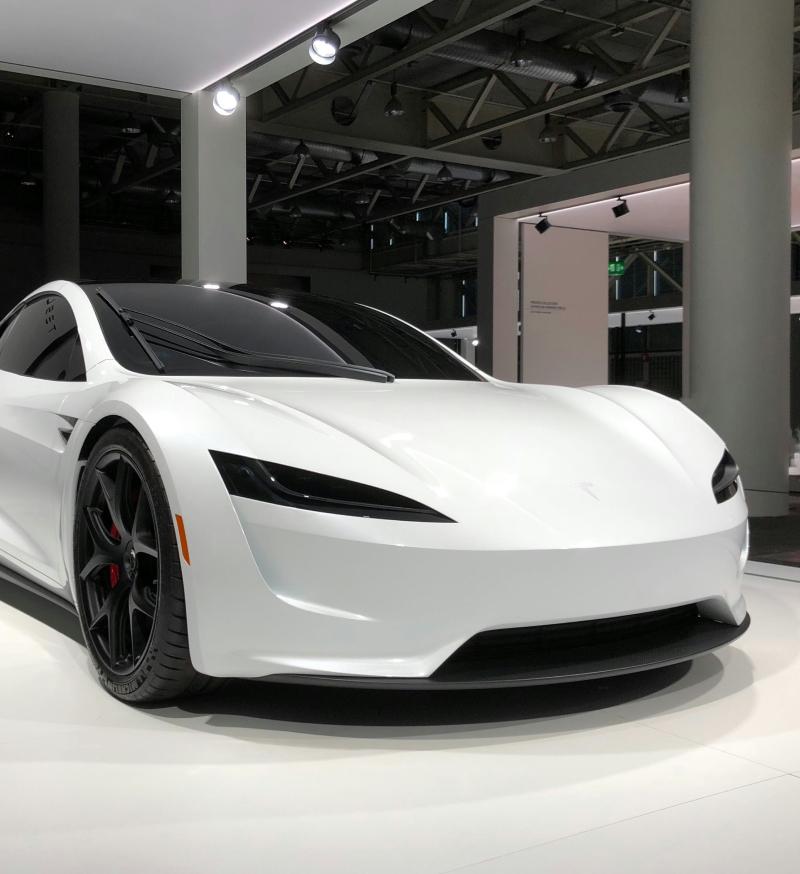
Gigafactory Texas, Austin, Texas, US
A dominant player, Tesla has become a leading innovator in electric vehicles and autonomous driving technologies. Tesla’s groundbreaking autopilot feature, building automation system (BAS) system, and smart Summon have positioned Tesla vehicles as the most advanced semi-autonomous systems available to consumers today.
LiDAR sensors, computer vision, artificial intelligence of things (AIoT), natural language processing, ML.
Tesla plans to launch its self-driving technology internationally in late 2024.
Tesla’s innovative EVs and autopilot technology have triggered the shift toward automated transportation solutions.
Tesla uses a combination of AI and machine learning in autonomous vehicles to enable various self-driving features, such as automatic lane keeping, traffic-aware cruise control, and autonomous navigation on highways.
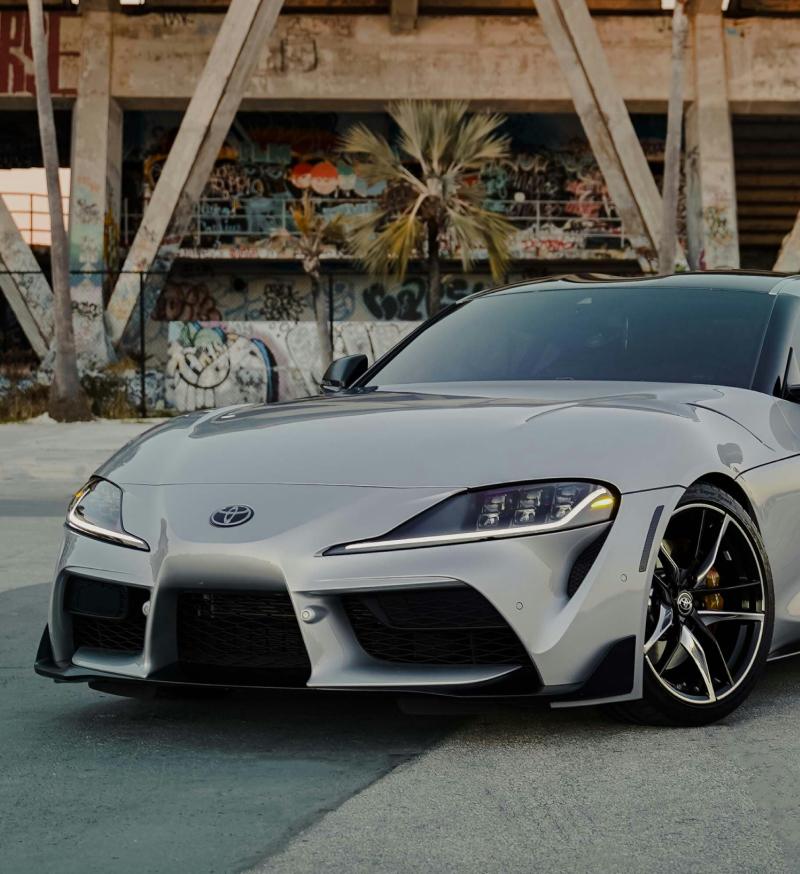
Toyota, Aichi, Japan
Toyota is making strides in developing AI-driven mobility solutions through its Research Institute and partnerships.
LiDAR and radar sensors, computer vision, AIoT, ML.
Toyota is working on its operating system for safety system monitoring and traffic monitoring.
Toyota’s Mobility Teammate Concept highlights the importance of augmented driving and human control over autonomous vehicles.
Toyota uses AI in autonomous driving as the backbone technology for its Chauffeur and Guardian driver assistance systems, elevating incident prevention capabilities.
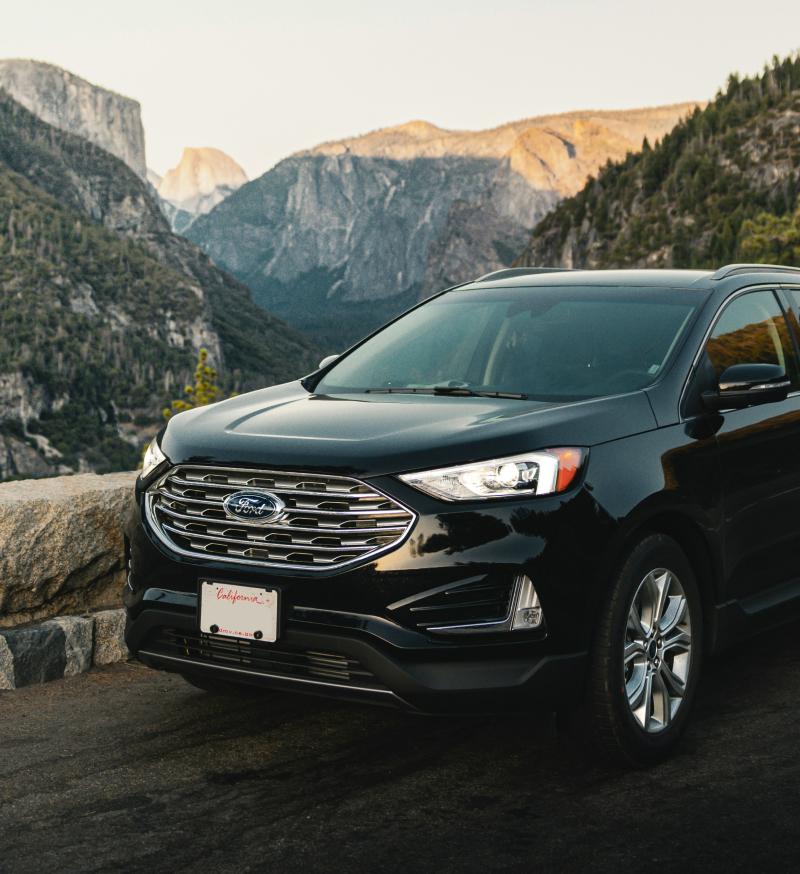
Dearborn, Michigan, USA
With a vast portfolio of automated driving technology, Ford has focused on Latitude AI, a subsidiary dedicated to developing a hands-free, automated driving system for commercial vehicles.
LiDAR and radar sensors, computer vision, AIoT, and ML.
Ford launched autonomous ride-hailing and delivery services backed by its proprietary tech.
Ford’s driverless cars and trucks are shaping the future of the transportation and delivery industries.
Ford leverages AI paired with LiDAR, radar, and infrared sensors to enable lane-keeping systems and adaptive cruise control. The company has also collaborated with companies like Argo AI to advance its AI capabilities.
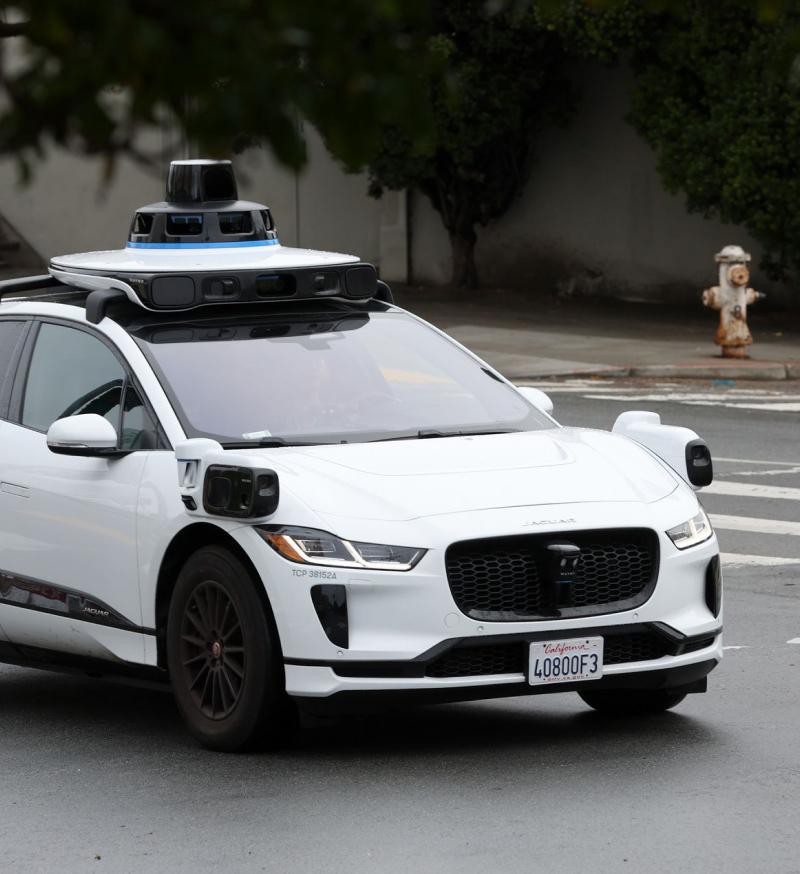
Mountain View, California, USA
Formerly known as the Google Self-Driving Car Project, Waymo branched off into Alphabet’s subsidiary focused solely on autonomous technology.
LiDAR and radar sensors, computer vision, AIoT, ML and neural networks.
Waymo Driver is an autonomous driving technology with one of the market's most advanced trajectory prediction capabilities.
Waymo has improved risk assessment methodologies and approaches to evaluating the safety of autonomous vehicles.
Waymo Driver, Waymo’s driverless technology, builds on a deep-learning architecture to predict vehicle trajectories in complex traffic scenarios and model vehicle interactions.
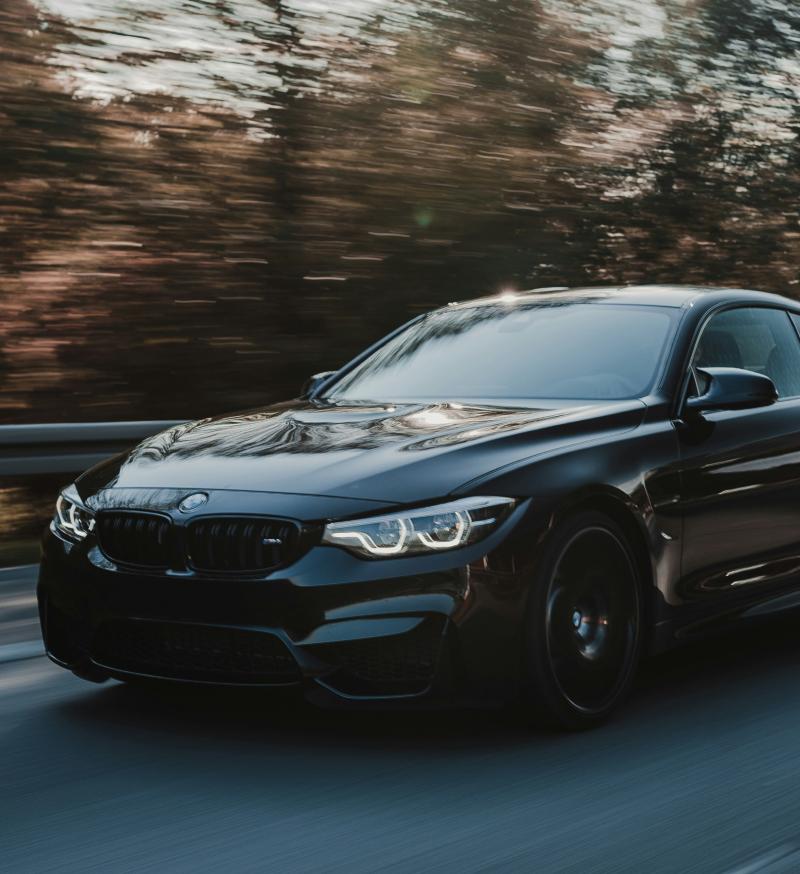
Munich, Germany
In the autonomous driving race since the 1990s, BMW has recently unveiled its Level 3 technology, which is now part of its 7 Series cars.
3D LiDAR and radar sensors, computer vision, AIoT, ML, and conversational AI.
The BMW Group is the first carmaker to offer both an advanced Level 2 driving function and a Level 3 system in its product portfolio.
BMW has been helping to create a future-proof standard for the safety of autonomous vehicle technology.
BMW relies on a blend of AI to enhance its driver assistance system, improve the comfort and safety of the occupants, and monitor the environment around the car.
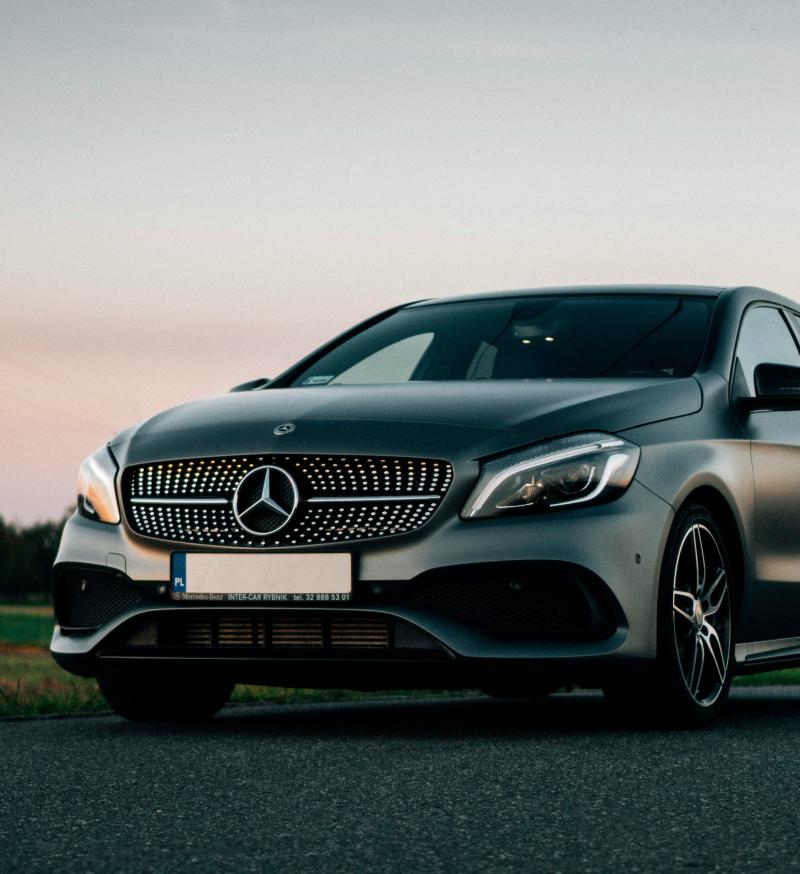
Stuttgart, Germany
Mercedes-Benz Group is another heavy hitter in autonomous vehicle development. It aims to implement advanced autonomous systems in its S-Class and EQS vehicle lines.
3D LiDAR and radar sensors, computer vision, AIoT, ML, and conversational AI.
Mercedes-Benz is the only car manufacturer with an internationally valid certification for conditionally automated driving.
Backed by its state-of-the-art driving assistance system, Mercedes-Benz Group has ushered in a new era of autonomous driving experiences.
Challenges of AI in autonomous driving
Although a handful of leading OEMs were able to push a few AI driving initiatives forward, machine learning in self-driving cars is far from reaching its peak potential. Most commercial projects never see the light of day, as the race toward a driverless future is fraught with numerous challenges inherent to the field.
Technological complexity and reliability
Differences in hardware and software specifications, the lack of standard protocols for automotive software development, and the difficulty in integrating diverse data from multiple sensors make automotive software development particularly challenging. Developing fail-safe deep learning algorithms adds complexity, as intelligent models must be fine-tuned for various conditions and environments to ensure real-time, reliable decision-making.
Advancements in AI research have contributed to the sharper perception, more intelligent decision-making, and enhanced reliability of AI algorithms. However, applying AI for autonomous vehicles still requires extensive testing and validation, including simulation and real-world trials, to ensure reliability and performance under diverse conditions.
Safety and security concerns
Autonomous vehicles rely heavily on ADAS and AD systems, which depend on software to ensure safe operation. A single sensor malfunction can compromise these critical systems. Additionally, the high connectivity of driverless vehicles increases the attack surface, allowing hackers to potentially take control of essential vehicle systems via wireless connections.
Addressing the system safety challenge calls for a comprehensive framework incorporating the FMEA approach, robust software security mechanisms such as redundant systems and authentication, and adherence to ADAS standards and safety protocols like SAE, ISO 26262 ASIL, and EURO NCAP. To ward off hackers, automotive software must be developed using state-of-the-art security technologies and receive regular software updates.
Regulatory and legal issues
While the global groundwork for higher penetration of ADAS systems has been laid, regulation coverage for completely autonomous systems remains insufficient. For semi-autonomous systems, variations and gaps in global regulations have left regulatory bodies unprepared for the unique challenges of autonomous vehicles.
To tackle the formidable challenge of industry regulation, technology developers, lawmakers, and global regulatory bodies must collaborate to create standardized, transparent, and fair rules that address the needs of all stakeholders. The industry also urgently needs a legal framework that defines liability and encourages innovation while protecting public safety.
Public acceptance and trust
Many incidents involving autonomous vehicles have raised doubts about safety and caused fluctuating consumer acceptance. Many consumers don't share OEMs' excitement about autonomous cars, often citing the unclear and unpredictable decision-making processes and the lack of transparency around sensors, mapping systems, and other driverless technologies.
One way to ensure consumer uptake is to establish transparency and tighter rules regarding the development of AVs. Public demonstrations, user testing, and educational campaigns will also help raise awareness about the benefits of AVs and move them from the dealership into consumers’ homes and hearts.
How Vention puts you in the first lane of the autonomous future
Custom AI solution development
- Tailored AI algorithms for autonomous driving applications like perception and decision-making systems
- Sensor fusion systems that integrate data from LIDAR, radar, and cameras to provide a comprehensive view of the environment
Simulation and testing
- Advanced simulation tools for testing AI models in virtual environments, encompassing rare and critical driving scenarios
- Supporting the setup and management of real-world trials to validate AI systems under actual driving conditions
Data management and analytics
- Assistance with acquiring, optimizing, annotating, and labeling diverse datasets for AI model training
- Automation of data collection and cleaning
- Implementation of analytics platforms to monitor AI performance and generate insights for continuous system improvement
Integration and system optimization
- Integration of AI software with vehicle hardware, ensuring smooth operations and real-time processing.
- Optimization of AI systems for efficiency, balancing performance with vehicle computational constraints
Training and support
- Technical training on the latest AI technologies and best practices in autonomous driving solution development
- Ongoing support and maintenance to ensure AI systems dynamically adjust to changes and learn from experiences
Why us?
Years of experience developing automotive solutions
Weeks from contact to kickoff
Engineers with AI-specific skill sets
AI projects successfully finished
Delivery on time, on budget, and on scope
Assistance in choosing stacks that reduce both upfront and ongoing maintenance costs
Our AI pros boast notable achievements in Kaggle competitions and projects
We’re an ISO 27001-certified company
Championed by the industry
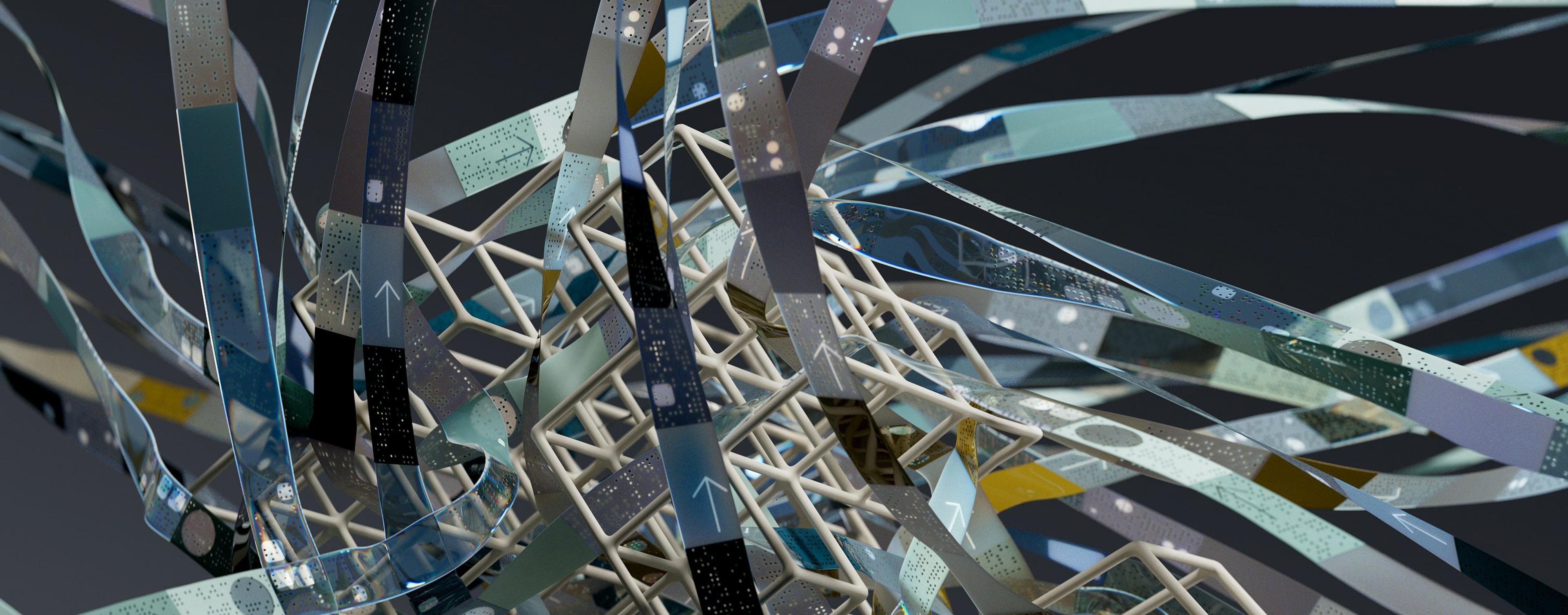
Need help with your autonomous driving project?
Let Vention be the engine behind your market success.